- Témaindító
- #1
- Csatlakozás
- 2024.09.10.
- Üzenetek
- 41,569
- Reakció pontszám
- 8
- Díjak
- 5
- Kor
- 37
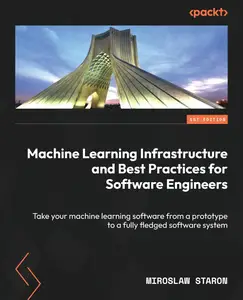
Free Download Machine Learning Infrastructure and Best Practices for Software Engineers: Take your machine learning software from a prototype to a fully fledged software system by Miroslaw Staron
English | January 31, 2024 | ISBN: 1837634068 | 346 pages | PDF | 24 Mb
Efficiently transform your initial designs into big systems by learning the foundations of infrastructure, algorithms, and ethical considerations for modern software products
Key FeaturesLearn how to scale-up your machine learning software to a professional levelSecure the quality of your machine learning pipeline at runtimeApply your knowledge to natural languages, programming languages, and imagesBook Description
Although creating a machine learning pipeline or developing a working prototype of a software system from that pipeline is easy and straightforward nowadays, the journey toward a professional software system is still extensive. This book will help you get to grips with various best practices and recipes that will help software engineers transform prototype pipelines into complete software products.
The book begins by introducing the main concepts of professional software systems that leverage machine learning at their core. As you progress, you'll explore the differences between traditional, non-ML software, and machine learning software. The initial best practices will guide you in determining the type of software you need for your product. Subsequently, you will delve into algorithms, covering their selection, development, and testing before exploring the intricacies of the infrastructure for machine learning systems by defining best practices for identifying the right data source and ensuring its quality.
Towards the end, you'll address the most challenging aspect of large-scale machine learning systems - ethics. By exploring and defining best practices for assessing ethical risks and strategies for mitigation, you will conclude the book where it all began - large-scale machine learning software.What you will learnIdentify what the machine learning software best suits your needsWork with scalable machine learning pipelinesScale up pipelines from prototypes to fully fledged softwareChoose suitable data sources and processing methods for your productDifferentiate raw data from complex processing, noting their advantagesTrack and mitigate important ethical risks in machine learning softwareWork with testing and validation for machine learning systemsWho this book is for
If you're a machine learning engineer, this book will help you design more robust software, and understand which scaling-up challenges you need to address and why. Software engineers will benefit from best practices that will make your products robust, reliable, and innovative. Decision makers will also find lots of useful information in this book, including guidance on what to look for in a well-designed machine learning software product.Table of ContentsMachine Learning Compared to Traditional SoftwareElements of a Machine Learning Software SystemData in Software Systems - Text, Images, Code, FeaturesData Acquisition, Data Quality and NoiseQuantifying and Improving Data PropertiesTypes of Data in ML SystemsFeature Engineering for Numerical and Image DataFeature Engineering for Natural Language DataTypes of Machine Learning Systems - Feature-Based and Raw Data Based (Deep Learning)Training and evaluation of classical ML systems and neural networksTraining and evaluation of advanced algorithms - deep learning, autoencoders, GPT-3Designing machine learning pipelines (MLOps) and their testingDesigning and implementation of large scale, robust ML software - a comprehensive exampleEthics in data acquisition and management
Buy Premium From My Links To Get Resumable Support,Max Speed & Support Me
Code:
⚠
A kód megtekintéséhez jelentkezz be.
Please log in to view the code.